And the Era of the Individual
“Claims that cannot be tested, assertions immune to disproof are veridically worthless, whatever value they may have in inspiring us or in exciting our sense of wonder.”
— Carl Sagan, The Demon-Haunted World: Science as a Candle in the Dark
Takeaways:
- We aren’t using position/motion tracking tech in Strength Coaching because it doesn’t exist (yet)
- Position/motion tracking seems to be the logical conclusion to sensing the training metrics that most strongly correlate to outcomes/wins
- Motion Based Training is poised to be as powerful in Strength Coaching as just about any other analytics system in sport
In competitive sports, the most important metric at the end of the day is a simple binary — did you win, or did you lose? This unforgiving fundamental nature is liberating in a way. Excuses, beliefs, and feelings don’t matter, what matters is objective truths we all share and experience, because when the dust settles there is either victory or loss.
The impact of sports’ inherent objectivity on the way we train athletes is similarly straightforward. If a system or methodology produces results it will survive the test of time. In their preparation for the Olympic Games, coaches in ancient Greece believed harmony in movement was integrally important to training success, so they would make sure there was at least one flute player at each practice, often synchronizing the flow of training with the melody. While music still plays a prominent role in training, I’m not familiar with any high profile programs that still employ flutists.
As the strength and conditioning world ebbs and flows over time, picking up a few ideas and technologies, leaving a couple behind, the filtering mechanism of wins and losses moves us slowly closer to what is optimal. Something worthy of note in this optimization algorithm is the simple fact that our industry can only create methodologies around tools for which a technology exists, and it can only employ methodologies for tools that are logistically useful. Put another way, if a technology doesn’t exist to, for example, train under increased gravity, then we cannot build the systems around it to integrate the technology into our workflow.
Compounding the impact of this fact is the reality that paradigm shifting technologies are rarely created specifically to help teams win games, but rather are borrowed from other industries. Viewed through this lens, we can start to understand how the role of technology in Strength and Conditioning got to where it is today. Heart rate sensors, strain gauges, even GPS and GLONASS technology existed in other forms before they were training tools. If we look at emerging technologies like Computer Vision, Narrow AI, Additive Manufacturing, etc., we can try to envision the future landscape of strength tech.
I’ll be focusing on two kinds of innovation: the improvement in quality or reduction in cost of existing systems or tools, and the creation or invention of completely new technologies. They are not mutually exclusive, in fact one is usually accompanied by the other. The main purpose of the differentiation is to highlight two key areas of focus of strength tech in the near future.
Motion Based Training
Our industry can only create and employ methodologies around tools for which a technology exists. That statement reads as obvious, and I don’t mean to sound as if I’m relaying a gem of wisdom I’ve mined over the past few years. I say it because the idea is a useful tool in figuring out why we train athletes with technology the way we do.
Take for example Velocity Based Training. Velocity acquisition devices have been around for decades. In fact one of the most basic patents for linear position transducing in an exercise application is set to expire soon. Louis Simmons is credited for introducing use of the Tendo FitroDyne to strength training back in 2002 in a Powerlifting USA article. If you take a look at the search interest in ‘velocity based training’, it took years after that for it to start gaining consistent momentum.
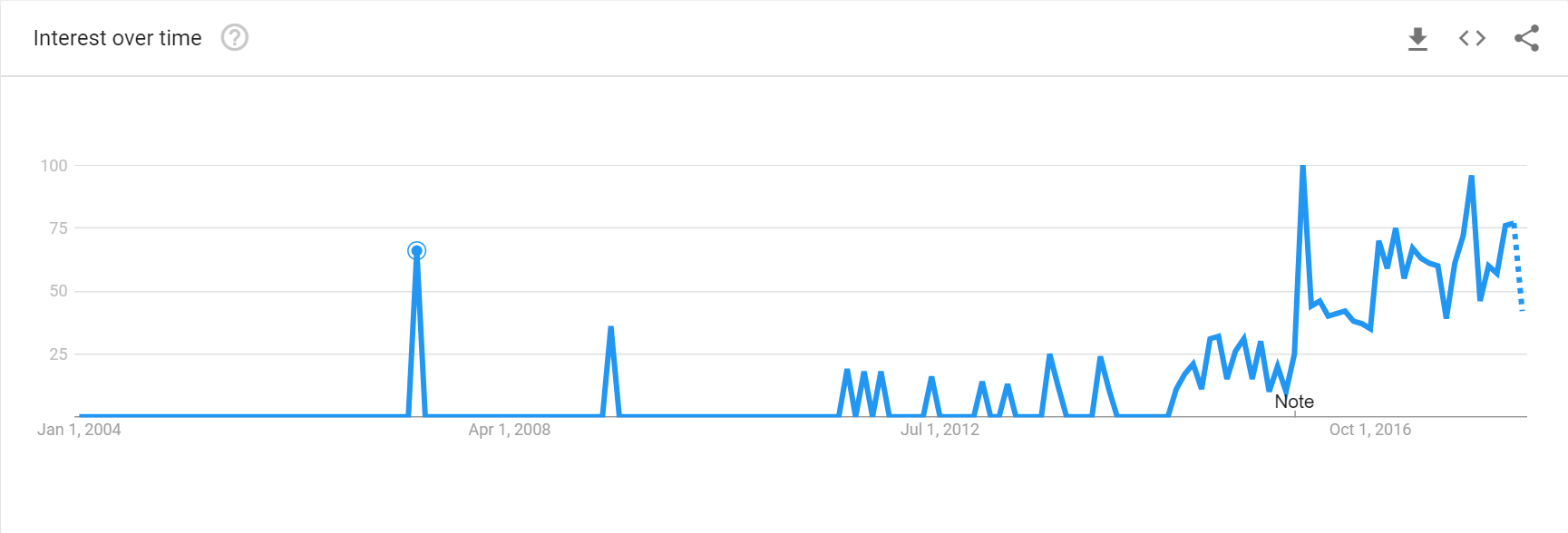
This data aligns with our desire back in 2013 to introduce low-cost, easy to use VBT devices. The technology was created, the methodologies were developed, tools became more accessible and easier to implement, and now velocity as a training metric is common language in strength.
Even though we’ve invested a lot of time and effort into VBT, we’ll be the first to mention its limitations. Autoregulatory measures like velocity and RPE vary on an individual basis, but it has proven quite difficult to figure out what factors are involved in this variability. There is a clear relationship between mean concentric velocity and RPE, but accurately predicting 1RM with velocity across populations is not such an easy task (which is why our tool is so flexible). That isn’t to say velocity and other autoregulation techniques are ineffective. They work quite well and can be impactful in myriad of ways. So where is this information gap coming from?
Data accuracy and subjective measures
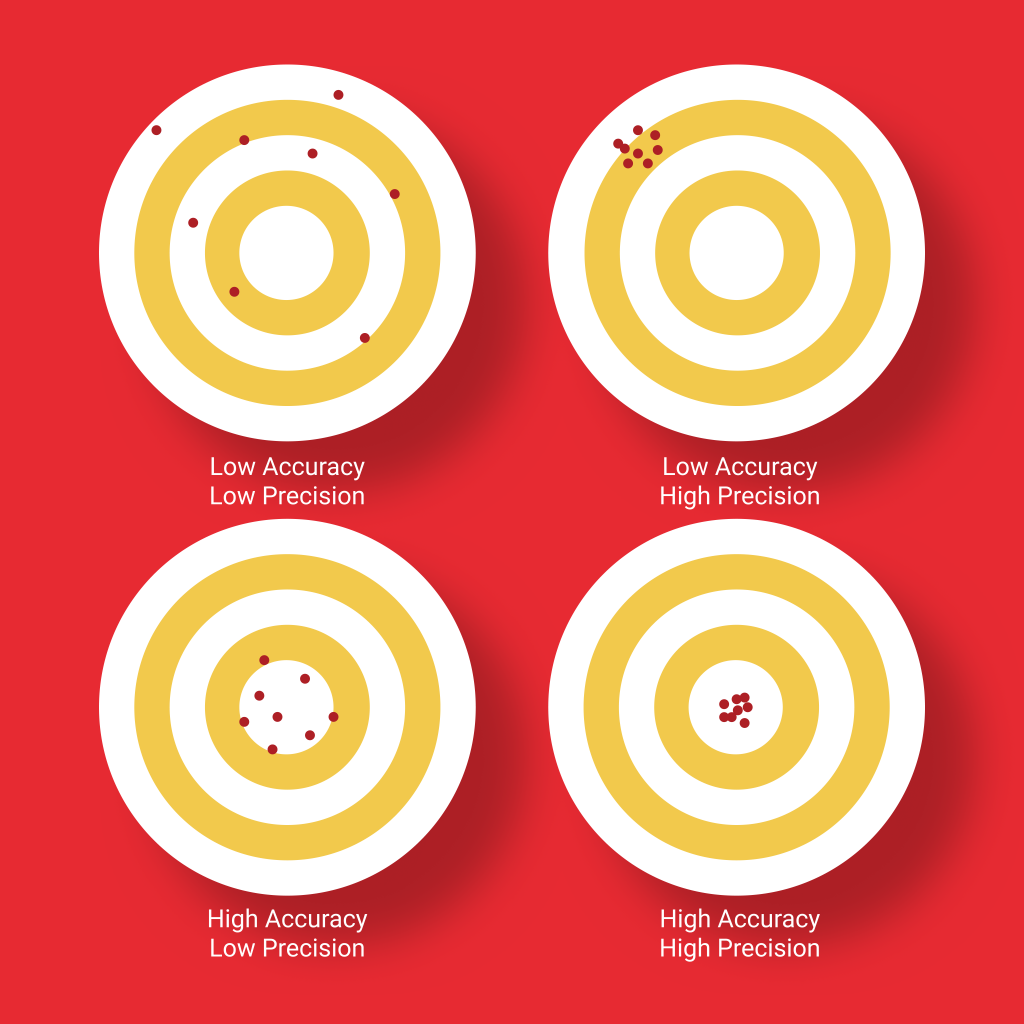
Data accuracy is incredibly important. Unbeatable accuracy has been a top priority of ours since we started building VBT products and will be for the foreseeable future. In the beginning of our quest for absolute accuracy we figured out a big problem in velocity tracking in general. Although our precision was high, and our accuracy within the constraints of a linear position measurement were very high, the real-world accuracy of one-dimensional measurement in general left quite a bit to be desired.
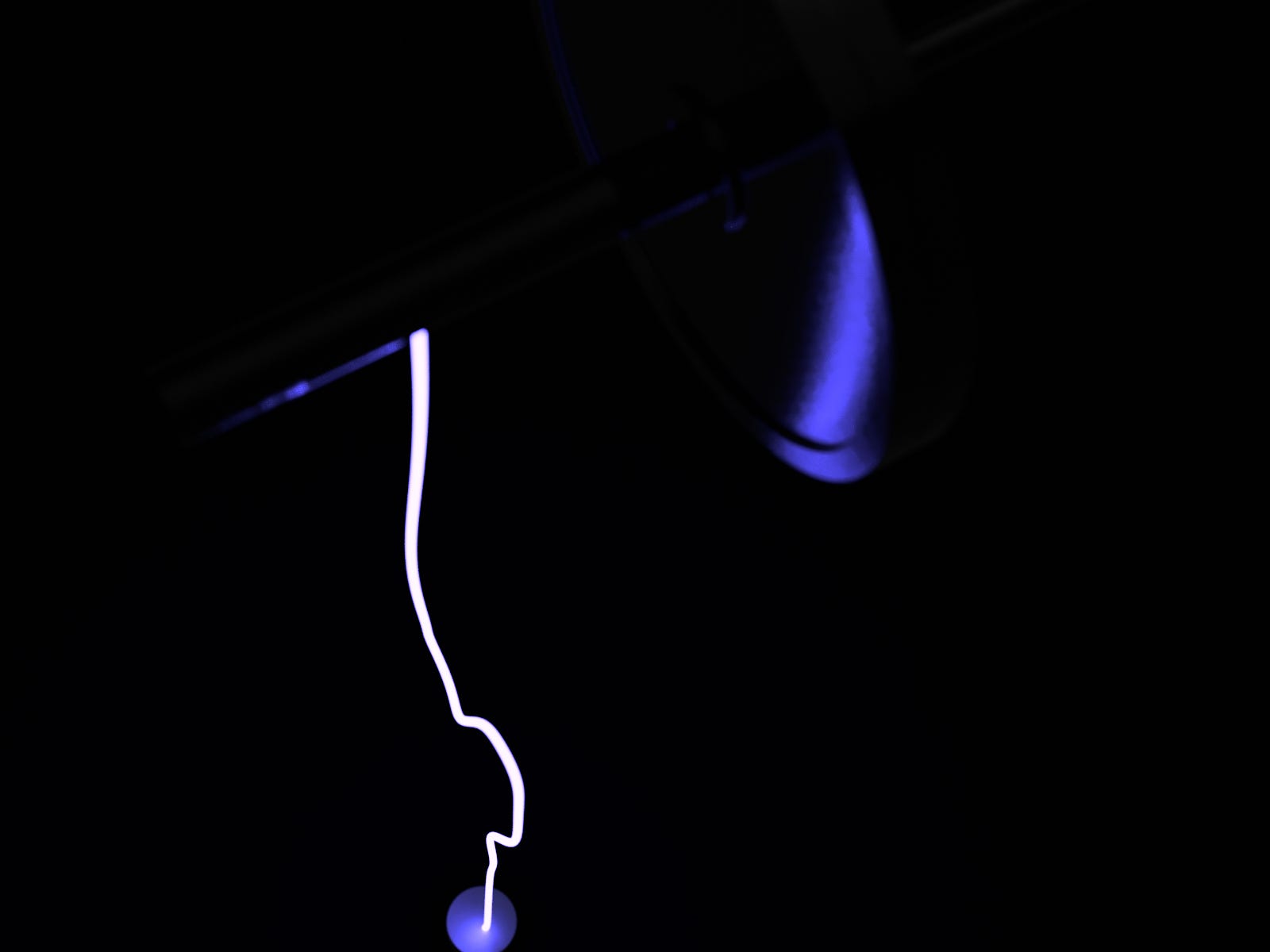
Our teaser remained a mystery to a surprising number of people (it’s a snatch bar path)
Humans, which constitute the majority of professional athletes, do not move in one dimension. They don’t even move in two dimensions. Every time you’ve ever moved it was through three spacial dimensions, and any mathematical model or lesser dimensional sensor will only be able to produce, at best, a close approximation of the real world movement you just did. To be fair, even a three dimensional sensor will only be able to produce a close approximation, but the ceiling on the accuracy and precision of a position measurement goes up significantly with such a device.
I’ve heard the argument that accuracy takes a backseat to precision in measurement tools for sport, because as the argument goes, as long as the measurements are repeatable then it’s good enough. This is inaccurate enough to be a topic for another blog post. If a tool is precise and not accurate, and its measurements are repeatable across several devices and consistent enough to be comparable to other users, then in the top right situation in our accuracy/precision picture above, we could just shift the results every time by the same amount and the tool would then be both accurate and precise. There’s a reason that’s not realistic.
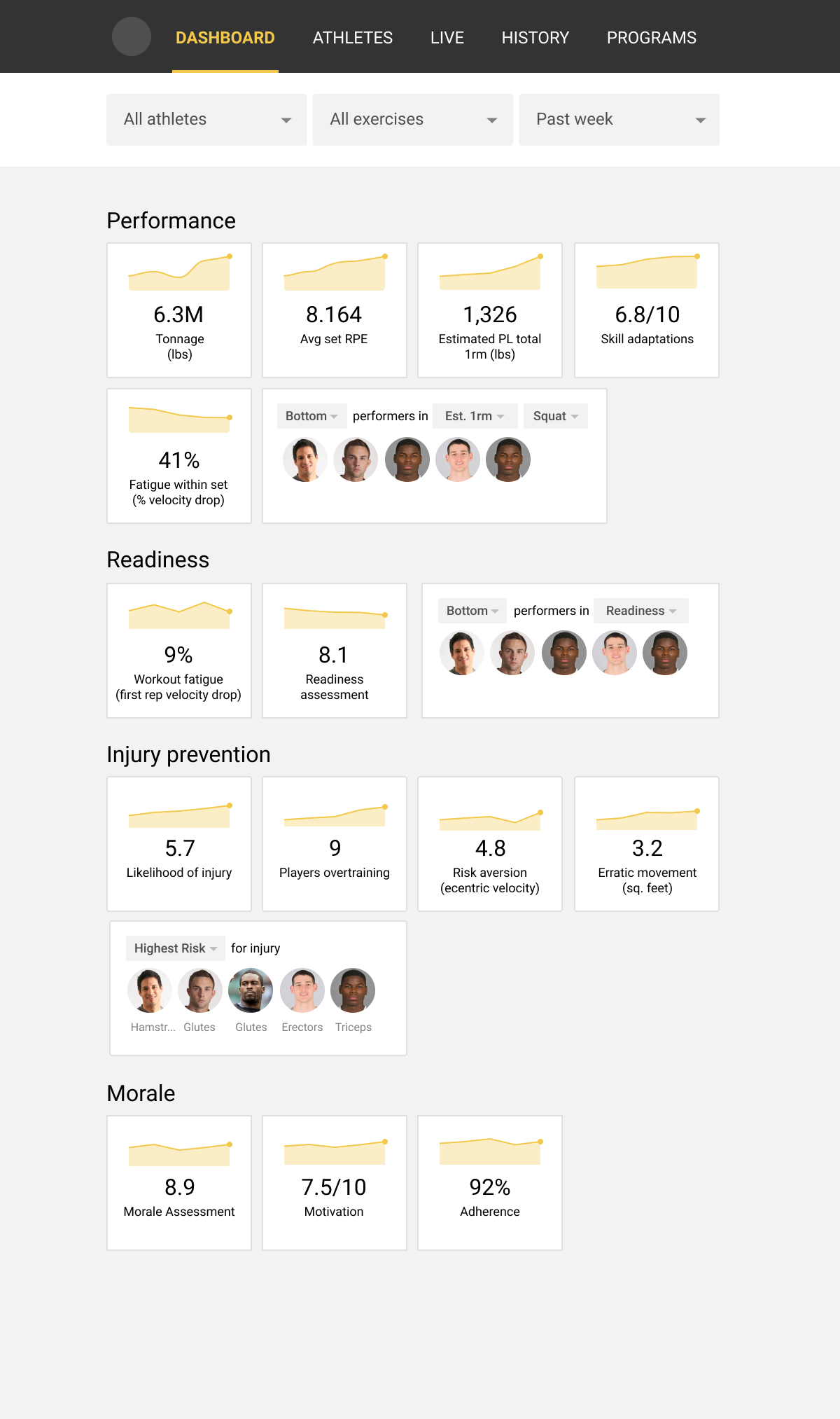
That being said, the utility of 3D position sensing in strength training goes far beyond increasing accuracy and precision. Once we can ascertain the real-world position of an object (or a point on an object) and can tie it to an athletes identity over a duration of time, we can do some seriously interesting stuff. Everything from skill adaptations (object path analysis) to inter and intra-set fatigue, overall readiness, likelihood of injury (erratic movement, lateral movement, abrupt pattern changes, etc.), risk aversion (eccentric velocity and subjective measures), and much more, can be tracked. This is enough content for an entire series of articles to come and gets to the core of the Motion Based Training approach.
Some of these qualitative metrics can only be measured in conjunction with subjective data, like morale and motivation. Collection of that data, usually in the form of athlete readiness assessments, has been growing in popularity for a good reason. It can be easyto administer to athletes, has been shown to be valid, and measures like subjective well being can be a powerful predictive tool for performance.
Tracking fatigue can be just as valuable, or even more valuable, than tracking velocity in strength sports. In a conversation I had with Travis McMaster of the University of Waikato in New Zealand, he mentioned how his velocity transducers are utilized nearly exclusively for the purpose of fatigue tracking.
Beyond our intuitive understanding of the matter, current research shows how closely change in velocity is correlated with fatigue in strength training. We also see signs in our own data that align with what’s known about self-reported motivation and its correlation to athlete burnout. The relationship to what an athlete reports via RPE, what we measure with velocity, and the athlete’s motivation and rate of overtraining and burnout, are great subject matter for future research.
The Physics Is in Our Favor
The cornerstone of the Motion Based Training approach is the idea of taking velocity for granted. Strength coaches are fortunate for several reasons, and high on the list is the fact that physics and mathematics loves weight training. The duration of a rep/set is brief, the displacement is short, net work is zero, inertia makes measurement easier with increasing weight, and the list goes on. If a position measurement tool is accurate enough and precise enough, it would make sense that basic movement variables could be highly predictive. If that tool is also easy enough to use and scalable to an entire team, we can enable coaches to make training decisions with a depth of knowledge that is rivaled only by the most advanced sports analytics technologies.
So why isn’t 3D position sensing currently utilized in strength training? The simple answer is that it doesn’t yet exist in consumer electronics. High spacial resolution position sensing is incredibly difficult even in high price, mass market products. We’ve evaluated technologies like Inertial Measurement Units (IMU’s, AKA accelerometers), ultrasonic sensors, IR range finders, Ultra Wide Band sensors, altimeters, GPS, and many others, only to find out what Apple, Samsung, and Facebook already knew — we weren’t going to find it. True high fidelity wireless position sensing likely won’t trickle down into a sensor we could simply plug into our product for several more years. When it eventually does, there’s no guaranteeing it’ll be accurate enough, scalable enough, easy enough to use, or affordable enough for use in strength.
We figured this out years ago and began working on specialized technology to accomplish exactly what we need. It took dozens of prototypes to get the sensing to where it is today, and we think it’s exactly what coaches need to enable Motion Based Training. We’ll talk a little more about our novel tech at a later date, and we’ll be working with several third parties to validate the output of our sensing.
The Future of Strength Tech
I’ve been asking coaches for a little while now what they think the weight room of the future will look like. In each response there is some aspect of movement sensing. Usually tablets on each training station, and an intelligent system that can interpret the deluge of data extracted from athletes. In fact there are some great companies in sports tech who share a similar vision of the future, and a lot of our customers have already outfitted their racks with tablets for things like session timing and workout logging.
Another clear trend we hear in these predictions is automation. Coaches tell us they want access to a ton of advanced metrics that they can “interpret quickly” with “no data analysis team needed”. A few of our customers are private sports training facilities who’s clients pay top dollar to access some of these futuristic training methods right now. The limitation is the expense of the analysis, delayed feedback, and coach-to-athlete ratio. In order for this kind of service to become the new normal in the weight room of the future, the data analysis needs to be automated so coaches can access simple reports and decisions can be made within minutes, or even seconds after the data is collected.
Even after coaches review our website and sign up for updates, they still imagine the above-described future weight room as being 10 to 20 years away. This is for good reason since, for the reasons described above, there has been no significant advances toward that future for decades. The concept of Motion Based Training isn’t meant to be a phrase adopted by the ‘industry’ or used as a replacement for any other ideas. The phrase is just a tool we’ve been using to communicate to coaches that the weight room of the future isn’t as far away as they think. In fact, we think it’s right around the corner.
If any of this sounds interesting to you, head over to RepOneStrength.com and sign up to stay in touch. If you want to help kickstart the Era of the Individual, simply share this article to Strength and Conditioning coaches or people influencers in the space. We’ll be sending email updates out periodically in the coming weeks in months as we head towards pre-orders this summer.