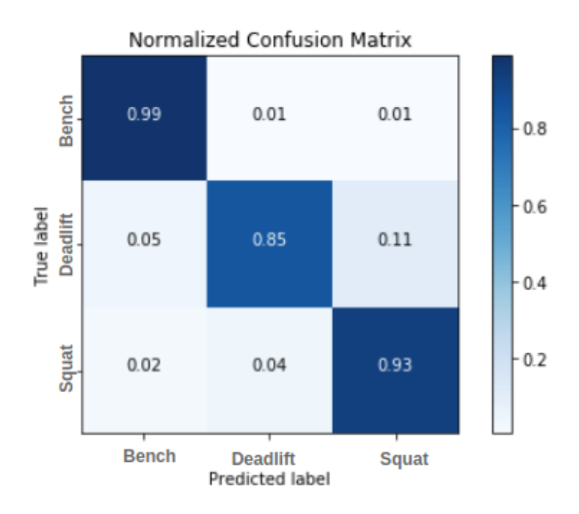
There are some amazing things developing in S&C…
A few of which we’ve summarized in our blog post about what we call ‘Motion Based Training’. Innovations that help us make sense of the training information that surrounds us will increasingly become paramount to success. Sports tech has been focused primarily on lagging indicators for years and products have gotten great at measuring and tracking in-game performance, but the time spent in-game is a small fraction of the time an athlete is performing. The relative lack of leading indicators has many reasons, not least among them is a lack of powerful data science.
In the effort of being proactive about our coaching rather than reactive, we need to be as far upstream as possible, which means collecting many pieces of data that can impact an athlete on game day. Recording meaningful data is a challenge, as devices like RepOne are only starting to deliver the necessary technology to teams, but once that barrier is cleared, making sense of it is the real challenge.
Lifter Clustering
This is a project we’ve been looking forward to since we started collecting lifting data. Lifter Clustering is the process of grouping athletes by specific traits in order to infer differences in performance with greater accuracy.
“What if we could analyze all the lifters in our database, determine natural groupings based on their performance metrics and create multiple versions of the [velocity calibration tables] for each distinct lifter profile?”
Lifter Clustering takes a non-traditional approach to exercise research, as it relies on a very non-traditional data source. The average strength study consists of something like 10 or 20 participants and lasts 4–6 weeks, where anything longer or bigger becomes exponentially more time intensive and costly. Where randomized controlled trials are highly supervised and precise, the OpenBarbell database is comparatively immense and longitudinal.
For example, rather than running a controlled study on the impact of long arms on bench press 1RM progress in beginner athletes, we look up the reverse. What were the ROMs on bench that correlated to low, moderate, and fast strength gain? Were there other factors that made longer armed lifters more likely to increase their strength? Were there a confluence of factors? Finally, if we observe these factors in a completely separate data set, do we have the same findings?
As our database increases in size, number of tracked metrics, types of metrics, and surrounding environmental data (limb lengths, sleep duration, etc.) we can start making sense of how the wide variety of factors that impact training work together to set PR’s.
Exercise Classification
Exercise classification is just as much for us as it is for you. A good chunk of our database is unclassified, which means we can’t currently use a sizable amount of our overhead press data because there’s no way for us to know that it is indeed an overhead press. It’s also useful during your workouts, as an athlete that logs less can focus more on their training. Once workout programs are uploaded to the RepOne Coaching Portal the system knows what an athlete is doing, but if he/she goes off program, we can get them back on course without requiring athlete input.
Initially we didn’t think one dimensional position data could tell us enough to infer exercise type, but our talented data scientist Yulia found some promising avenues. This isn’t brand new tech, but executing exercise classification at the accuracy our customers require is quite different than what your Apple Watch can do. As 3D sensing becomes more prevalent these estimations will become more capable, leading to features like genuinely useful automated form critique.
Have you taken our ‘How Do You Coach’ Surveys?
If you haven’t, you’re missing out on a chance to win a free RepOne device. Everybody who fills out all of our surveys is eligible to win one of the most advanced training tools in the world. Check the links below!